Data Labeling: The Backbone of AI and Machine Learning
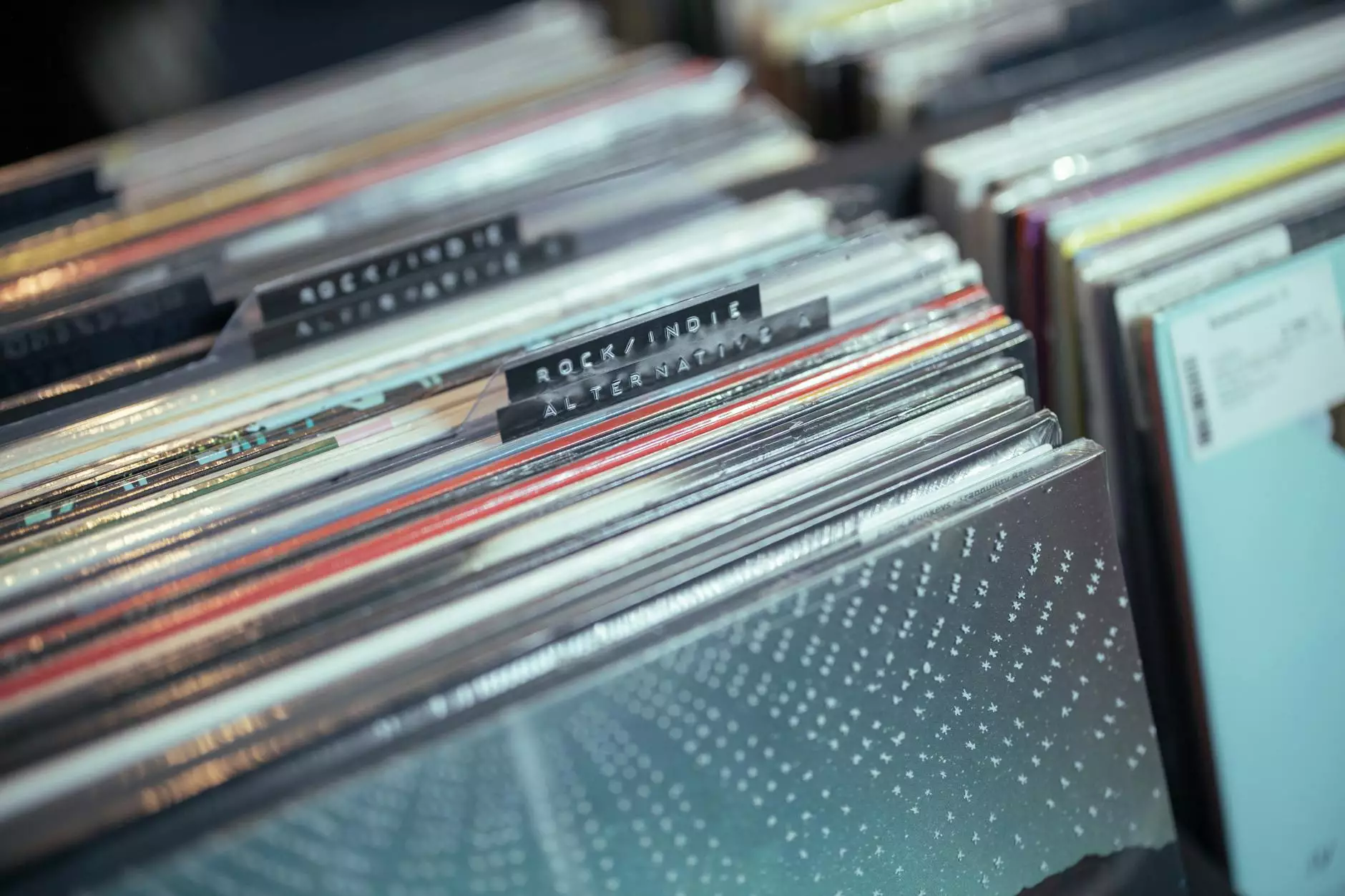
Data labeling has emerged as a crucial component in the field of artificial intelligence (AI) and machine learning (ML). As businesses increasingly harness the power of AI technologies, the significance of data labeling continues to grow. In this article, we will explore the intricacies of data labeling, its impact on software development, and how companies like Keymakr are leading the way in this dynamic field.
Understanding Data Labeling
At its core, data labeling involves annotating or tagging data with relevant labels that facilitate the training of machine learning models. This process transforms raw data into a structured format that algorithms can understand and learn from. The labeled data serves as a foundation for model training, enabling AI systems to make accurate predictions or classifications.
The Importance of Data Labeling
Without proper data labeling, machine learning algorithms cannot function effectively. Here are several reasons highlighting its significance:
- Improved Model Accuracy: Labeled data allows models to learn patterns and relationships in the data, leading to higher accuracy in predictions.
- Efficient Learning: Proper labeling accelerates the learning process, reducing the time and resources required for training AI models.
- Enhanced Understanding: Data labeling provides researchers and developers with insights into the data, helping to refine algorithms and improve outcomes.
- Quality Control: Well-labeled datasets reduce the likelihood of errors in AI systems, increasing their reliability and robustness.
The Data Labeling Process
The data labeling process can be broken down into several key steps:
1. Data Collection
Before labeling can occur, businesses must collect relevant data. This data can come from various sources, including:
- Surveys and questionnaires
- Audio and video recordings
- Text documents and articles
- Images and graphics
2. Defining Labeling Guidelines
Clear guidelines must be established to ensure consistency in labeling. This includes specifying what labels will be used and how they should be applied across different data types. Detailed instructions help maintain accuracy and uniformity throughout the labeling process.
3. Manual or Automated Annotation
Depending on the project's scale and complexity, data labeling can be performed manually by human annotators or through automated systems, such as machine learning algorithms designed for the task. Each method has its pros and cons:
- Manual Annotation: Offers high accuracy and can capture nuances that machines might miss, but it is time-consuming and resource-intensive.
- Automated Annotation: Works quickly and efficiently but may require human oversight to correct inaccuracies.
4. Quality Assurance
After the data labeling is completed, a rigorous quality assurance process is essential. This step involves reviewing and verifying the labeled data to ensure it meets the established guidelines. Techniques used may include:
- Cross-validation between different annotators
- Statistical analysis on labeling accuracy
- Spot checks for random samples of data
5. Dataset Preparation
Once quality checks are complete, the labeled data can be formatted and structured for use in machine learning models. This preparation ensures that data is ready for training, validation, and testing phases of model development.
Applications of Data Labeling in Business
The applications of data labeling in the business landscape are vast and varied. Here are some key areas where labeled data plays a crucial role:
A. Natural Language Processing (NLP)
Businesses employing NLP technologies rely heavily on labeled text data for tasks such as sentiment analysis, text classification, and entity recognition. By accurately labeling large datasets of text, businesses can develop AI models that understand and generate human language.
B. Computer Vision
In the realm of computer vision, data labeling is fundamental. Applications such as image recognition, object detection, and facial recognition require meticulously labeled image datasets. Industries like automotive (for self-driving vehicles) and security (for surveillance) are prominently using labeled data to train their models.
C. Healthcare
The healthcare industry utilizes data labeling to develop AI systems that can accurately diagnose diseases from medical images or patient data. Labeled datasets enable models to learn from past cases and improve the effectiveness of diagnostics tools.
Challenges in Data Labeling
While data labeling is essential, it is not without its challenges. Some of the common issues faced by businesses include:
- Scalability: As the amount of data grows, so does the need for efficient labeling solutions that can scale without sacrificing accuracy.
- Cost: Manual labeling can be labor-intensive, resulting in significant costs for businesses. Balancing quality and expense is a critical concern.
- Consistency: Ensuring that all annotators apply labels consistently is challenging, particularly when dealing with subjective categories.
- Complexity of Data: Certain types of data, such as multimedia inputs, may require specialized knowledge for accurate labeling.
Keymakr: Pioneering Data Labeling Solutions
Keymakr exemplifies excellence in the field of data labeling. Recognized for their innovative approach, they offer a suite of high-quality labeling services tailored for various industries:
Customized Solutions
Keymakr provides personalized labeling solutions that adapt to the unique requirements of each client. Their experienced annotators work closely with businesses to ensure that the labels are relevant and actionable.
AI-Enhanced Efficiency
Utilizing a blend of human expertise and machine learning, Keymakr streamlines the labeling process. Their hybrid approach not only enhances speed but also maintains high levels of accuracy.
Robust Quality Assurance
Quality is a cornerstone of Keymakr's operations. With rigorous validation processes and continuous improvements, they ensure that clients receive only the best-labeled data for their projects.
Conclusion
In conclusion, data labeling is an indispensable aspect of modern AI and machine learning applications. It empowers businesses to harness the full potential of their data, driving innovation and efficiency. As the demand for high-quality labeled datasets continues to rise, companies like Keymakr stand out as leaders, providing tailored solutions that meet the diverse needs of industries around the globe. By investing in top-notch data labeling services, businesses can unlock new opportunities and remain competitive in an increasingly data-driven world.
Final Thoughts
As we move forward, it's essential for businesses to recognize the importance of data labeling in their strategic planning. The right labeled datasets will lead to better AI models, more informed decision-making, and ultimately, greater success in their respective fields. Let us embrace the power of data labeling and pave the way for a future driven by intelligent technology!